Understanding Generative AI: Revolutionizing Creativity and Innovation
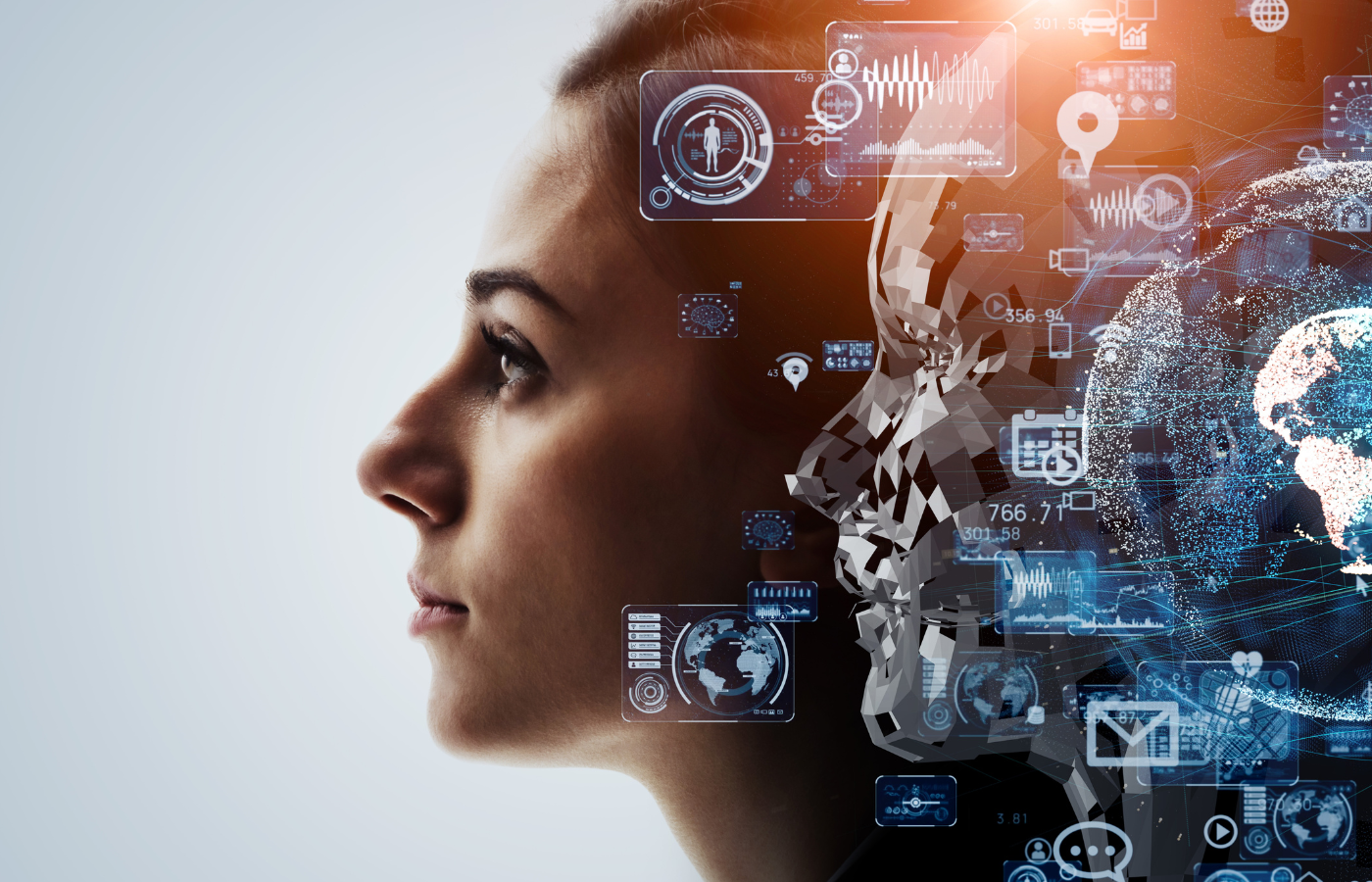
Table of Content
- Introduction
- How Does Generative AI Work?
- Key Applications of Generative AI
- Advantages of Generative AI
- Challenges and Ethical Considerations
- The Future of Generative AI
- Conclusion
What is Generative AI?
Generative AI refers to a class of artificial intelligence systems capable of producing new content rather than simply analyzing existing data. Unlike traditional AI, which follows pre-programmed instructions, generative AI can generate text, images, music, and even video content by learning patterns from large datasets. This is done using sophisticated models like neural networks, especially deep learning architectures.The most well-known example of generative AI is GPT (Generative Pre-trained Transformer) by OpenAI, which powers advanced language models, enabling machines to understand and generate human-like text. Other well-known models include DALL·E for image generation and MusicLM for music composition.
How Does Generative AI Work?
Generative AI relies on machine learning techniques, particularly unsupervised learning or semi-supervised learning. Here's a breakdown of its components:
- Data Collection and Training: Generative AI models are trained on vast amounts of data, whether it be images, text, or audio. This training allows the model to understand underlying patterns and features in the data.
- Neural Networks: Deep learning models, particularly transformers and GANs (Generative Adversarial Networks), are commonly used in generative AI. These models consist of layers of neurons that mimic how the human brain works, learning patterns and generating outputs based on input data.
- Generative Process: Once trained, generative AI can produce content by using the learned patterns to predict and create new data. For example, a model like GPT can generate coherent text based on a prompt, while a model like DALL·E can generate images from textual descriptions.
- Fine-Tuning: Often, these models are fine-tuned on specific datasets to achieve better results for niche applications, such as creative writing, code generation, or even designing video game characters.
Key Applications of Generative AI
Generative AI is transforming industries across the board. Here are some key sectors where it is making an impact:
- Content Creation:
- Text Generation: Models like GPT can write articles, essays, and creative stories. They are even used for customer service chatbots and content marketing.
- Image and Video Creation: Tools like DALL·E and MidJourney can create stunning visuals from simple text prompts, revolutionizing the world of art and design.
- Music Composition: AI models like MusicLM create music by analyzing patterns from existing compositions and generating new melodies.
- Healthcare:
- Drug Discovery: Generative AI can assist in identifying new compounds for drugs by analyzing molecular structures.
- Medical Imaging: AI can generate synthetic medical images for training models, improving diagnostic accuracy in radiology.
- Software Development:
- Code Generation: Generative models can assist in writing code, reducing the time developers spend on routine tasks. GitHub Copilot is a great example, where developers get AI-assisted code suggestions.
- Gaming and Entertainment:
- Character and World Design: AI can generate characters, environments, and entire storylines, making it an invaluable tool in game development and animation studios.
Advantages of Generative A
- Efficiency: Generative AI can automate the creation of content, whether it’s writing code, designing graphics, or generating new product designs. This leads to improved productivity and cost savings.
- Personalization: AI can create highly personalized experiences, such as generating custom content, recommending products, or crafting personalized learning experiences.
- Scalability: With generative AI, it’s easier to produce large-scale content. For example, content marketing strategies can leverage AI to generate blog posts, social media content, and video scripts quickly.
- Creativity Augmentation: Generative AI doesn’t replace human creativity; it enhances it. Artists, writers, and designers can use AI as a tool to explore ideas and push creative boundaries further.
Challenges and Ethical Considerations
Despite the remarkable potential of generative AI, there are challenges and ethical concerns to consider:
- Bias in Data: AI models are trained on data that may contain biases, which can lead to biased outputs. It's essential to ensure diversity in training datasets to avoid unintended discrimination.
- Misinformation: AI can generate highly realistic fake news, deepfakes, or misleading content, raising concerns about trustworthiness and authenticity in media.
- Intellectual Property: The content generated by AI may sometimes closely mimic existing works, leading to potential copyright issues.
- Job Displacement: As generative AI automates creative tasks, there is concern over job displacement in fields like content creation, design, and even software development.
The Future of Generative AI
Generative AI is still in its infancy but is evolving rapidly. With ongoing research and development, we can expect more sophisticated models capable of tackling complex problems across various domains. As it matures, the key focus will likely be on addressing ethical concerns, improving transparency, and ensuring responsible use.
Conclusion
Generative AI is more than a technological trend; it's a fundamental shift in how we approach creativity, problem-solving, and automation. As businesses and industries continue to explore its potential, it’s clear that the future will be shaped by the symbiotic relationship between human creativity and AI-powered innovation. Whether it's writing stories, composing music, or solving scientific problems, generative AI holds the key to unlocking new frontiers of imagination and progress.